By Shaojun Gui & Tak-San Ho
Efficient and accurate characterization of the time evolution of many-body systems controlled by external fields is of fundamental importance to many applications such as laser-driven chemical reactions and quantum computing. A full-dimensional description of such many-body dynamics is prohibitively expensive when large number of subsystems (e.g., spins in a spin-chain and atoms in a large molecule) are involved due to the exponential increase of the system state space dimensionality.
Our project develops and implements new discrete real-time learning methods based on the state of the-art Artificial Neural Network (ANN) machine learning (ML) technique for solving large many-body dynamics, aiming at making possible accurate large-scale quantum optimal control simulations beyond the existing studies that have been primarily limited to small quantum systems.
To design optimal controls that drive the time evolution of many-body systems toward designated objectives, we synergize the ML technique with Quantum Optimal Control Theory (QOCT) to identify exponentially small effective spaces where the optimally driven dynamics is involved. We demonstrated the high versatility and efficiency of our synergistic ML-QOCT scheme in the control of local excitation in quantum systems consisting of strongly coupled particles including the 1D/2D Heisenberg spins and 2D coupled harmonic oscillators. In the future stage of the project, we will apply the synergistic ML-QOCT scheme to the selective excitation of chemical bonds in polyatomic molecules where many vibrational and electronic degrees of freedom are coupled.
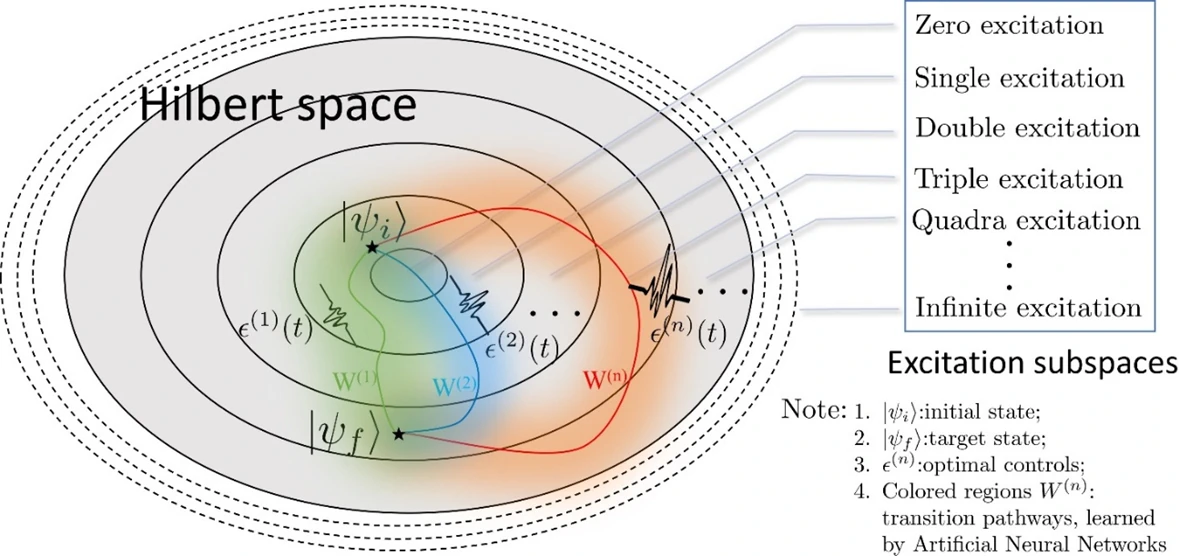
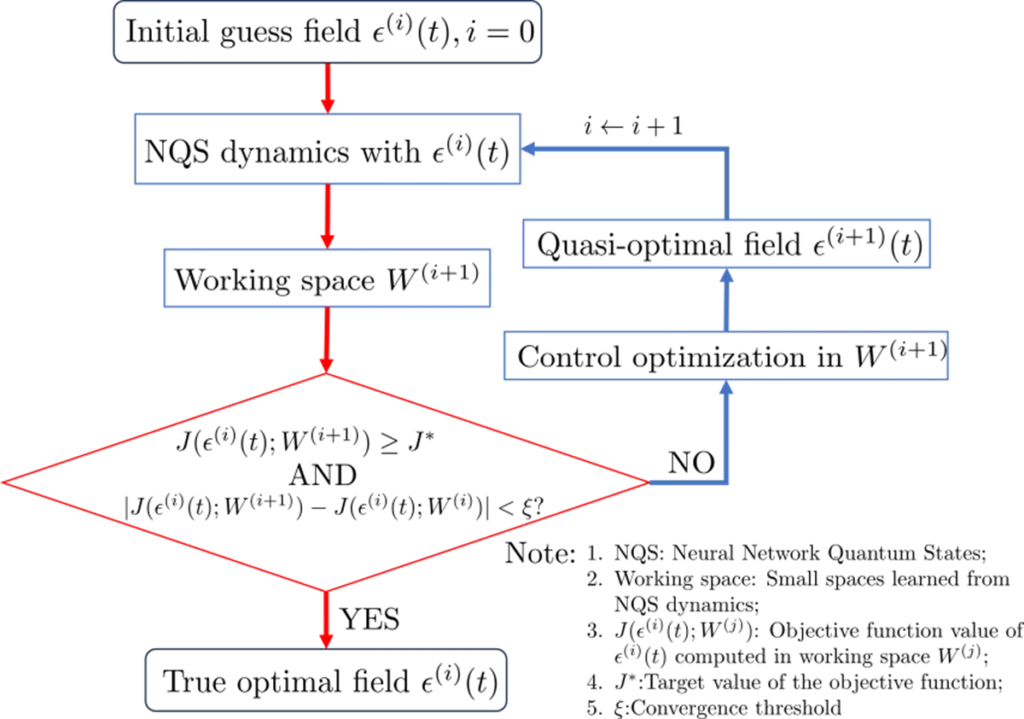